Date:2025/6/4 14:20-15:30
Location:R102, CSIE
Speakers:Prof. Kuan-Hao Huang
Host:林軒田教授
Abstract:
Large language models (LLMs) have shown remarkable potential in real-world applications. Despite their impressive capabilities, they can still produce errors in simple situations and behave in ways that are misaligned with human expectations, raising concerns about their reliability. As a result, ensuring their robustness has become a critical challenge. In this talk, I will explore key robustness issues across three aspects of LLMs: pure text-based LLMs, multimodal LLMs, and multilingual LLMs. Specifically, I will first introduce how position bias can hurt the understanding capabilities of LLMs and present a training-free solution to address this issue. Next, I will discuss position bias in the multimodal setting and introduce a Primal Visual Description (PVD) module that enhances robustness in multimodal understanding. Finally, I will examine the impact of language alignment on the robustness of multilingual LLMs.
Biography:
Kuan-Hao Huang is an Assistant Professor in the Department of Computer Science and Engineering at Texas A&M University. Before joining Texas A&M in 2024, he was a Postdoctoral Research Associate at University of Illinois Urbana-Champaign. His research focuses on natural language processing and machine learning, with a particular emphasis on building trustworthy and generalizable language AI systems that can adapt across domains, languages, and modalities. His research has been published in top-tier conferences such as ACL, EMNLP, and ICLR. His work on paraphrase understanding was recognized with the ACL Area Chair Award in 2023.
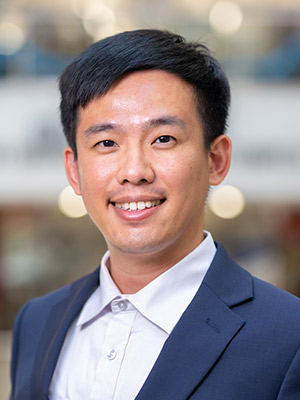